前言
Flux.1[dex] 是 Flux 系列中权重公开且效果最好的模型
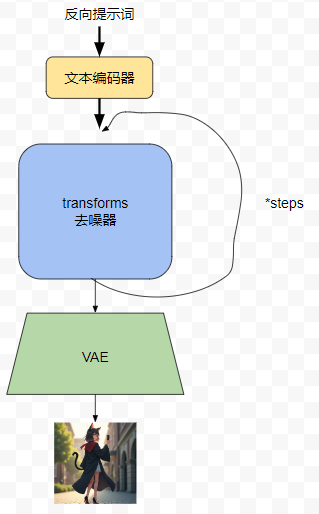
结构类似于 Stable Diffusion 3 ,其中的 transformer 模块是一个 12B 的模型,在没有量化的情况下是无法正常塞入一张小于 26G 显存的显卡的,在通过 diffusers 的 FluxPipeline.from_pretrained 加载时就是配置了 device_map 也只能把 transformer 放入 cpu 中运行。
cpu 运行速度对我们来说是万万不能接受的, transformer 模块作为去噪器运算量占据整个模型的百分之99以上。因此必须要手动分割才能完整加载入 gpu 中运行。
博主的机器配置为 4 x NVIDIA A10 (24G)没有 NVlink 。。。(如果有的话可以尝试其他并行方式)
模型划分
对于模型我们主要分成3部分处理
prompt 处理部分 --> Transformer 去噪部分 --> VAE 图像解码部分
class Flux_model:
def __init__(self):
self.promptpipeline = FluxPipeline.from_pretrained(
ckpt_id,
transformer=None,
vae=None,
device_map="balanced",
max_memory={0: "16GB", 1: "22GB"},
torch_dtype=torch.bfloat16
)
transformer = FluxTransformer2DModel.from_pretrained(
ckpt_id,
subfolder="transformer",
device_map=Transformer2DModel_device_map,
torch_dtype=torch.bfloat16
)
self.transformerpipeline = FluxPipeline.from_pretrained(
ckpt_id,
text_encoder=None,
text_encoder_2=None,
tokenizer=None,
tokenizer_2=None,
vae=None,
transformer=transformer,
torch_dtype=torch.bfloat16
)
self.vae = AutoencoderKL.from_pretrained(ckpt_id, subfolder="vae", torch_dtype=torch.bfloat16).to("cuda:0")
self.vae_scale_factor = 2 ** (len(self.vae.config.block_out_channels))
self.image_processor = VaeImageProcessor(vae_scale_factor=self.vae_scale_factor)
prompt 部分我们把它分在 cuda 0 ,1 上
VAE 我们简单放在 cuda 0 上
对于 Transformer 部分就要手动切分了,通过 device_map 参数我们设置
Transformer2DModel_device_map = {
"context_embedder": 2,
"norm_out": 3,
"proj_out": 3,
"single_transformer_blocks": 3,
"time_text_embed": 2,
"transformer_blocks": 2,
"x_embedder": 2
}
来保证在每一个扩散步骤中,只发生两次 GPU 之间的数据交换
推理
我们简单的把模型串起来
def __call__(self,
prompt:str,
prompt_2:str = None,
height:int = 1024,
width:int = 1024,
steps:int = 50,
):
with torch.no_grad():
print("Encoding prompts.")
prompt_embeds, pooled_prompt_embeds, text_ids = self.promptpipeline.encode_prompt(
prompt=prompt, prompt_2=prompt_2, max_sequence_length=512
)
print("Running denoising.")
# No need to wrap it up under `torch.no_grad()` as pipeline call method
# is already wrapped under that.
latents = self.transformerpipeline(
prompt_embeds=prompt_embeds,
pooled_prompt_embeds=pooled_prompt_embeds,
num_inference_steps=steps,
guidance_scale=3.5,
height=height,
width=width,
output_type="latent",
).images
print(latents.shape)
with torch.no_grad():
print("Running decoding.")
latents = FluxPipeline._unpack_latents(latents.to("cuda:0"), height, width, self.vae_scale_factor)
latents = (latents / self.vae.config.scaling_factor) + self.vae.config.shift_factor
image = self.vae.decode(latents, return_dict=False)[0]
image = self.image_processor.postprocess(image, output_type="pil")[0]
return image
便完成推理流程,模型初始化后占用,
|====================================+
| 0 NVIDIA A10 Off | 00000000:3B:00.0 Off |
| 0% 54C P0 62W / 150W | 5316MiB / 23028MiB |
+------------------------------------+-----------------------+
| 1 NVIDIA A10 Off | 00000000:5F:00.0 Off |
| 0% 52C P0 59W / 150W | 9660MiB / 23028MiB |
+------------------------------------+-----------------------+
| 2 NVIDIA A10 Off | 00000000:AF:00.0 Off |
| 0% 54C P0 59W / 150W | 13512MiB / 23028MiB |
+------------------------------------+-----------------------+
| 3 NVIDIA A10 Off | 00000000:B0:00.0 Off |
| 0% 54C P0 62W / 150W | 11370MiB / 23028MiB |
+------------------------------------+-----------------------+
绰绰有余
简单跑一跑
RAW photo, an intimate close-up of a slightly voluptuous blonde woman, lying in bed while kittens of all colors cuddle with her. The happiness in her expression is captured in stunning 8K resolution with photorealistic sharpness and detail, making the image a perfect, realistic masterpiece.
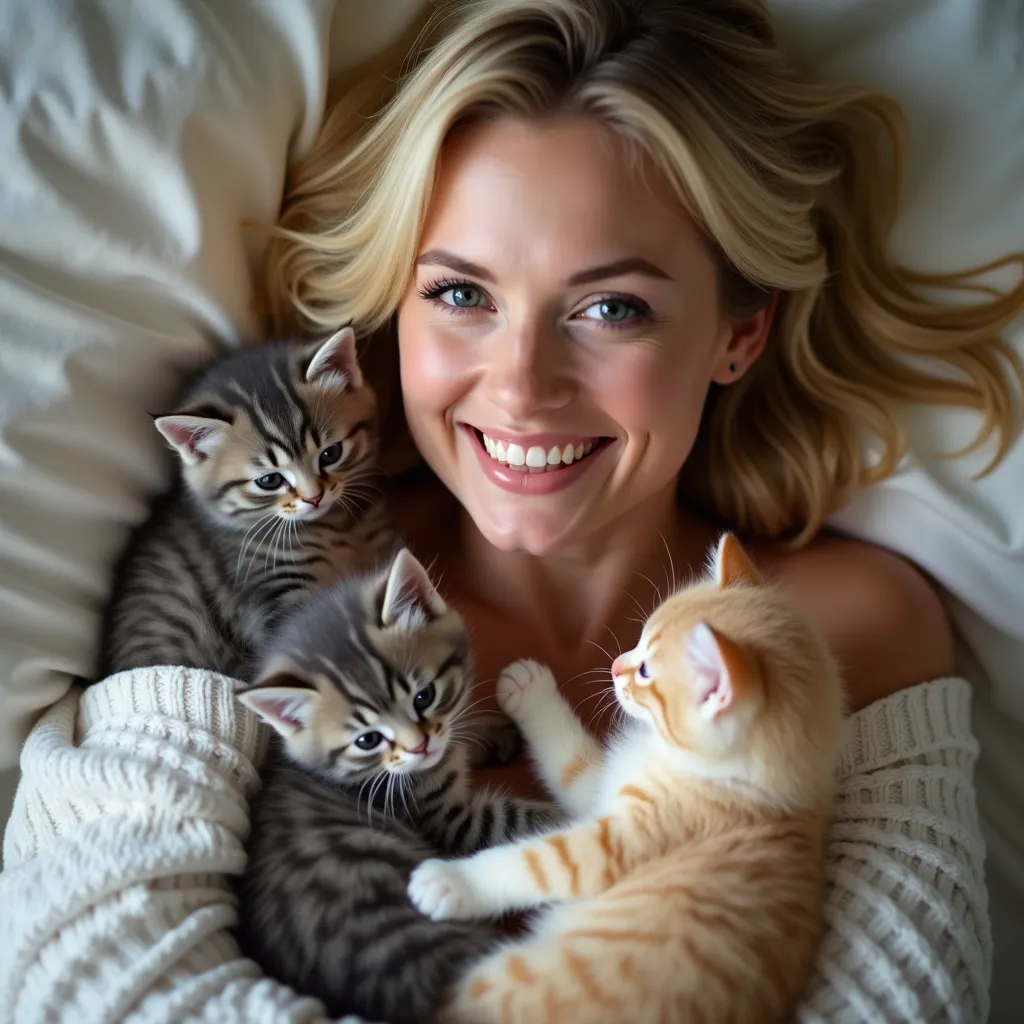
Comments NOTHING